Example of Predictive Analytics in the Insurance Industry - For a moment here I would like to focus an example of predictive analytics in the insurance industry, in the auto claim process. What we'd like to think about is what does it look like for the customer and for the claims handler? Will all of this analytics increase the level of red tape customers will have to jump through or will it increase the workload for claims handler? If we take a look at this example here, I'd like you to meet customer Michael Jones. Michael is a very busy father of two young children. He's out for a Sunday drive, and he just gets distracted and drives the car off the side of the road, and he needs help from his insurance company. What does the process look like for Michael and for the claim handler? In a nutshell this is Michael's experience. He rings up his insurance company. He tells them what happened. Sarah is the claim handler who is assigned to handle his case. She asks him some questions. His car gets fixed, and he's a happy customer. So that's all Michael sees, and he's happy at the end of the day. What about Sarah? What's the impact on her? Well, Sarah takes the call from Michael. She is prompted by the system to ask certain questions. She tells Michael where to bring a car to be fixed, and Sarah is also happy because she's confident that she had dealt with Michael efficiently and correctly. We have a happy customer, and we have a happy claims handler...
Example for Marketing Analysis - The second example was for marketing analysis in the field for very large automobile manufacturer. Their big objective as a company was to change their relationships with the dealers. Become more consultative with the dealers, more consultative in terms of what’s selling, what kinds of promotions work on which vehicles. Going down to the vehicle level, in fact even down to the version of those vehicles, including discounts on after market parts. The company wanted to push profit and loss responsibility down to the regional level and to do that the company had to provide regional sales managers with much greater visibility and much greater ability to explore their data. Sales reps and managers are able to quickly ask entirely new questions with an interface that has this walkup usability, and it actually gives them a greater ability to consult with the folks who run the dealerships themselves. We did a similar thing with another customer, a company that sells seeds, many different kinds of seeds. They have different properties engineered into the seeds, and this company uses test beds all over the country so that they can get really good data on the yield that different kinds of seed produce in different kinds of soil at different kinds of latitude...
Examples of Companies Using Analytic Scorecards - For example, a pharmaceutical company is trying to measure the relationship they have with key thought leaders and doctors. So they have a thought leader engagement index, they call it. They identify within each field who are the big thought leaders, who are people writing the books, writing the papers or are influential over other doctors and other academics. They’ve identified these top 15 or 20 people around the world, and then they measured the relationship they have with them. The highest level of relationship might be a doctor that will go to seminars for you and talk about the wonders of your product to other doctors. You’ve been working together for years and they have total trusting, friendly type relationship. Another doctor might be one that won’t even give you an appointment, and you’re still just communicating through emails and phone calls, and so you have the beginnings of a relationship there, but maybe they don’t trust your pharmaceutical company, and they’ve had bad relationships with you before, and so that would be an example of somebody you’re trying to get a first date with whereas the other one would be like a marriage...
Exploration and Analysis - With exploration and analysis we are really navigating through historical sets of data. It’s much more of a top-down type of analysis where we see the results. And then we have a hypothesis, a mental-model of perhaps of what caused that result, and then we go explore the data, drill-down to hierarchies across dimensions to find the root cause. So in that respect it is very deductive type of reasoning that we employ in that type of analysis. But, typically we are using query tools and OLAP tools to do that type of analysis...
Export Companies Use Analytics - In International trade, there are several trade blocks and International blocks present. These trade blocks often have specific rules and regulations that can change the statistics and the overall profits and losses of exports. To avoid these specific tools that can be termed analytics, keep track of the regular up-downs of international trade. How Do you Analyze Export Data? Trade Statistics Trade statistics is all about understanding the pattern of trade, trade policies of a particular country, and specific trade blocks in general. It is keeping track of various policies and programs introduced by the Trade blocks all over the world. It requires specific tools to analyze the strategic position of export conditions of the world. Individual companies can use these to diversify trade and adjust the sail towards them. Tax and tariffs in the market and market requirements are essential points to focus on. Market requirements tilt a country's production towards those goods, and tariffs help the market requirements stabilize. Using different market analysis maps, it is possible to understand these trends beforehand for a particular firm...
Faster Better Cheaper Analytics and Decision-Making - Okay well, faster, better, cheaper analytics and decision-making, these are the goals I see very often in many verticals. The stakes here and the scale here just seem to be much larger. So speaking of scale you have a fourth generation analytic solution. Tell us at the high level a little bit about that before we delve a bit more detail about the technological requirements. What is it that you've developed that helps deal with these issues of scale? Jim: So our platform really harnesses big data pipeline technology that makes it accessible to users in a very interactive and highly seamless in immersive environment will allows them to zero in on pieces of data. And the analytic tools allow them to make decisions very quickly and with very high quality confidence that the data is going to really help to make a high quality decision. So we bring large amounts of claims and other sources of data together, and we fuse that together into a very large data set, and we run a lot of machine learning analytics over the top of that and provide a variety of visualizations and navigational constructs for them. We allow them to see what the provider looks like in terms of how much money that they've been billing, what kinds of activities have they been doing, what relationships they have to other providers or other institutions and to really surface in a very visual way that interconnectivity that they had into a healthcare network or a plan...
Features of Good Financial Analysis Software - Financial analysis is the process of analyzing finances to identify and fix issues. To do this, you need good financial analysis software with the right features. Luckily, the market has several financial analysis software you can invest in for your business. What Is Financial Analysis Software? Financial analysis software is a tool that helps you analyze your business data and make decisions based on that data. This software isn't only for people doing accounting or finance work. Even if you're not an accountant, you can use it to understand your business better and make higher-quality decisions. Financial analysis software can be used as a standalone tool or integrated into an accounting or financial management system. In either case, it has many features that make it useful in helping you perform financial analysis tasks...
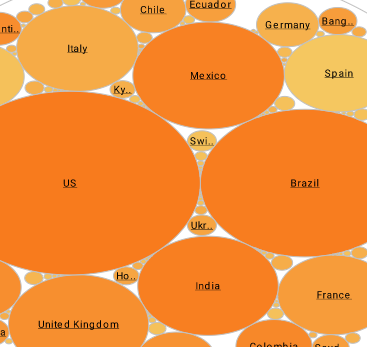 |
“Flexible product with great training and support. The product has been very useful for quickly creating dashboards and data views. Support and training has always been available to us and quick to respond.
- George R, Information Technology Specialist at Sonepar USA
|
Five Tips on How Analytics and Data Can Be Useful for e-Commerce Owners - In the early days of eCommerce, the first retailers had to overcome a great challenge of convincing customers to trust them. Giving out credit card numbers and personal details online was something that many didn't really trust, so Amazon and other retailers had to do a great job to get the public put their faith into this new way to pay and shop. As customers have gotten super comfortable with shopping online, the eCommerce industry proliferated, with global retail sales reaching $3.53 trillion in 2019. Today, online retailers have to face yet another profound problem: the diversity of customer choice. While this may not sound like an issue at all, giving too much choice and too little assistance and guidance can: easily confuse customers and make them leave the site convince them to put off the purchase look for a product on a competitor's site visit a brick and mortar store instead...
Food Safety Analytics - With the powerful analytics functions provided by InetSoft, a health inspection dashboard such as the one above can be generated to help local health departments keep track of food safety issues in different boroughs and different types of establishments. It can also provide local restaurant owners with improvement guideline on common issues, enabling them to prepare for inspection...
Four Types of Analysts - All right, so let’s meet your analysts. There are four types of analysts out there, and they have labels: “Super User”, “Business Analyst”, “Analytical Modeler”, and “Business Manager.” Super User, he is a business guy in sales, or marketing, or finance who has gravitated toward the technology, finds that he likes using Excel or the BI tools, likes building reports and rolling up his sleeves and wading into the data. And over time he has become the go-to guy for everyone in the department to build ad hoc reports or ad hoc views of information. He may not have report creation and design as part of his job description, but this is something that he had carved out a reputation doing. Next is the “Business Analyst” who was hired to crunch data on a day-to-day basis. She probably has an MBA, probably very good Excel skills, Access skills, may know a little bit of SAS, SPSS. She probably has a title of Financial Analyst, or Marketing Analyst, or Sales and Operations Analyst, and plays a lot of different roles within the organization...
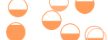 |
Read what InetSoft customers and partners have said about their selection of Style Report as their production reporting tool. |
Fraud Reduction in eCommerce with Better Analytics - Predictive analytics and big data are two ways eCommerce sellers can identify fraud, which is becoming a pressing issue. According to the Global Fraud Report 2018, almost three-quarters of online retailers (72 percent) agree that eCommerce fraud is a growing concern; moreover, 63 percent of them "have experienced the same or more fraud losses in the past 12 months." Before the arrival of data analytics tools, eCommerce sellers utilize a sample of customer data for fraud analysis. This means spending a lot of time and money to investigate the entire sample because the analysis would have to be manual. Now that big data analytics systems are available, retailers can analyze all data for fraud much quicker. One way to battle eCommerce fraud with data analytics is to use predictive analytics. Here are the steps involved in this process: Prepare the database of online orders from your store. This means defining a timeframe for orders Define the types of transactions to include in the database. Since eCommerce fraud happens only with credit cards, exclude orders where creating a chargeback is impossible Identify the patterns of fraudulent orders to differentiate between the good and the bad transactions Model the data to teach the algorithm to define suspicious orders based on the patterns. This is where data intelligence professionals and/or tools perform approaches like deep learning algorithms Implement the model Add new fraud patterns to the algorithm. As a result, it would be possible to reduce the amount of fraudulent orders by rejecting them and prohibiting fraudsters from making purchases...
Free Analysis Software - InetSoft provides users with a free visualization tool, Visualize Free. Based on the advanced commercial dashboard and visualization software, this software offers a Web-based solution that allows managers to interactively explore large, complex sets of data and represent them through visually-compelling graphics and charts. Visualize Free features various analytic components which are fully customizable to satisfy any company’s visualization requirements. Sets of data are modifiable to different graphical views enabling multi-dimensional analysis and allowing managers to observe specific growth trends or aberrations...