In this digital era where troves of data are being collected every second, it is important to utilize tools and techniques that help understand this data if it is to inform better business decisions. Machine learning is a branch of artificial intelligence that is being widely used in analyzing data using algorithms to learn from the data. It then makes intelligent suggestions and predictions based on the data collected.
Integrating data visualization with this data analysis technique brings in a suite of tools that help in finding a qualitative understanding of these massive amounts of data. Read on to understand how tapping into the possibilities of machine learning data visualization is important in improving data analytics.
#1 Ranking: Read how InetSoft was rated #1 for user adoption in G2's user survey-based index |
|
Read More |
Ensuring an efficient machine-learning process
By automating time-consuming operations such as data collection, information organization, and trend reporting, machine learning solutions can boost productivity and efficiency. All of those jobs, even with enormous amounts of data, may be completed instantly thanks to machine learning business applications. The machine learning process takes several steps, each of which can benefit greatly from data visualization. Using data visualization techniques, data scientists can focus on the broader picture of the data at each step rather than on scrutinizing values. This allows them to pay attention to model design, model performance, and the meaning of data. Here is how data visualization is useful in each step.
1. Putting data into context
Despite being simple to report and evaluate, raw data can be misleading when taken out of context. The machine learning process starts with asking relevant questions. This entails putting the data into context before setting the data up for modeling to get the whole picture of the data. What this means is applying data visualization tools and techniques to visualize every column with the data. The purpose of this step is to understand what each column of data means. Data scientists also seek to understand the type of variables for each column. Is it a continuous or a categorical variable or is it a dependent or independent variable? Putting the data in visuals makes it easy for the human mind to grasp and put it into context.
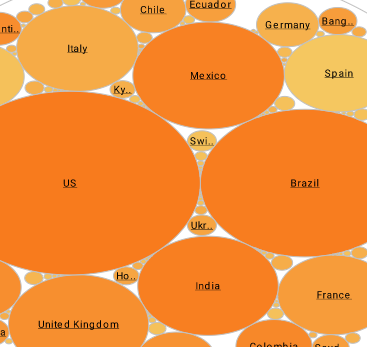 |
“Flexible product with great training and support. The product has been very useful for quickly creating dashboards and data views. Support and training has always been available to us and quick to respond.
- George R, Information Technology Specialist at Sonepar USA
|
2. Understanding the data
Data visualization plays an important role in understanding the data in depth. This step also involves asking and answering relevant questions such as where possible data errors or data imbalances are. Data visualization helps answer such a query by helping to identify the areas of data where there are possible errors such as outlier data, misleading data, missing data or wrong data types. If an error such as missing data is identified, data visualization tools can be used to determine the density of the missing data. In case of data imbalances, data scientists identify areas of high density for further investigation.
Additionally, data visualization helps understand how variables are related as well as how strong the relationships are. It can be used to show the meaning of particular data sets within a larger data set, providing a perspective on the data. By presenting data in a graphical or pictorial format such as graphs, maps, and charts, it becomes easy to comprehend correlations within massive data sets. Further, by applying knowledge graph machine learning in the process, data analysts can make predictions over complex, interrelated data. However, it is important to have clear data for data visualization. Cleaning data allows accurate data that yields reliable visualizations and models.
3. Model creation and tuning
Despite the fact that prior knowledge of the model and data may be useful, it might be difficult to determine the ideal parameters through experimentation. It would take a lot of time and processing resources to manually perform thorough searches for the ideal hyperparameters. For this reason, hyperparameter tuning that is automated is employed to find the best values. A typical tuning procedure involves changing a few hyperparameters, rerunning the algorithm on the data, and comparing its performance to that of your validation set to see which set of hyperparameters produces the most accurate model.
Model tuning enables you to alter your models so that they produce the most precise results and provide you with extremely useful knowledge and insight into your data, empowering you to take the best possible business decisions. During model tuning and model creation, advanced data visualization techniques can be used to make the process actual or concrete. For instance, a technique such as TensorFlow lets analysts follow the process of model creation with data visualization at every stage. In the process of building a TensorFlow model, data visualization can help visualize the structure and to follow the data to determine if it fits the design.
4. Making sense of the data
The purpose of machine learning is to help organizations make well-informed decisions. Making Sense of Data instructs readers on the procedures and concerns that must be taken into account in order to finish a data analysis or data mining project successfully. There are so many various angles to take when it comes to handling and analyzing data in order to obtain understanding. This is something that the entire field of statistical analysis routinely does. However, like with many other marketing-related tasks, mastering the fundamentals and a handful of specific tasks will usually suffice. At the end of the process, data analysts have a task to help executives and other users understand and make sense of the data in a way that it is easier to use the data for decision-making. Data analysts can use data visualization to present the facts drawn from the data in visual formats that are easy to understand. This can be done in a way that the visualization tells a data story that leads to conclusive decisions.
Conclusion
The purpose of machine learning is to draw conclusions from raw data that help in making data-backed decisions. Using data visualization techniques in the machine learning process eliminates the abstractness of the model creation and tuning process. It plays a vital role in helping analysts put the data into context as well as understand the data exhaustively. Most importantly, data visualization helps analysts present data in a way that users can understand it better.